Can you become a Machine Learning Research Engineer without a degree?
An alternative career path to becoming a Machine Learning Research Engineer with its major challenges, possible benefits, and some ways to hack your way into it.
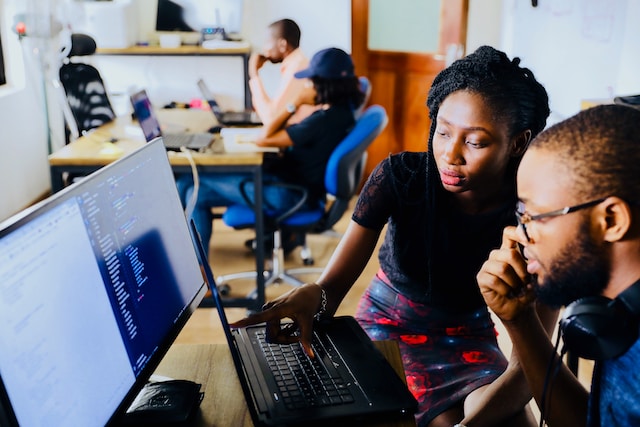
Yes, it is possible to become a Machine Learning Research Engineer without a formal degree. While a degree can provide a solid foundation in the field, it is not the sole determinant of success in this career path. Many successful professionals in the field have gained expertise through self-study, practical experience, and a strong portfolio of projects.
How to achieve this career goal without a degree?
-
Self-Study: Start by learning the fundamentals of machine learning and deep learning. Online platforms like Coursera, edX, and Udacity offer courses on these topics. Begin with introductory courses and gradually progress to more advanced topics such as neural networks, natural language processing, and reinforcement learning. Supplement your learning with textbooks and research papers to gain a deeper understanding of the subject matter.
-
Build a Strong Portfolio: Practical experience is crucial in this field. Work on personal projects that demonstrate your skills and understanding of machine learning concepts. Create projects that showcase your ability to solve real-world problems using machine learning techniques. Sharing your projects on platforms like GitHub or Kaggle can help you gain visibility and credibility.
-
Contribute to Open Source Projects: Collaborating on open source projects can provide valuable experience and help you learn from experienced professionals. Contribute to projects related to machine learning libraries, frameworks, or research. This not only enhances your technical skills but also demonstrates your ability to work in a team and contribute to the community.
-
Networking and Building Connections: Attend conferences, workshops, and meetups related to machine learning and artificial intelligence. Engage with professionals in the field, ask questions, and seek mentorship. Building a strong network can open doors to opportunities and provide guidance throughout your career.
-
Research and Publish: Engage in independent research and publish your findings. Participate in machine learning competitions or submit papers to conferences and journals. This demonstrates your ability to conduct research and contribute to the field.
Hacks and Advice
-
Create a Learning Plan: Develop a structured learning plan to ensure you cover all the necessary topics. Identify the areas you need to focus on and set goals for each topic. This will help you stay organized and track your progress.
-
Join Online Communities: Engage with online communities such as forums, subreddits, and social media groups focused on machine learning. Participating in discussions, asking questions, and sharing knowledge can help you learn from others and stay updated on the latest trends and techniques.
-
Stay Updated: Machine learning is a rapidly evolving field. Stay updated with the latest research papers, industry trends, and new tools and frameworks. Follow influential researchers and industry leaders on platforms like Twitter and LinkedIn to stay informed.
Difficulties and Benefits
While pursuing a career in machine learning without a degree is possible, it does come with some challenges. Some potential difficulties include:
-
Lack of Formal Credential: Without a degree, you may face initial skepticism from employers who prioritize formal education. However, a strong portfolio and practical experience can help overcome this hurdle.
-
Structured Learning: Self-study requires discipline and self-motivation. Designing a structured learning plan and sticking to it can help overcome this challenge.
On the other hand, there are several benefits to pursuing a career in machine learning without a degree:
-
Flexibility: Without the constraints of a formal degree program, you have the freedom to explore various topics and focus on areas that interest you the most.
-
Cost Savings: Pursuing a degree can be expensive. Self-study allows you to acquire knowledge and skills at a lower cost.
-
Hands-on Experience: By working on personal projects and collaborating on open source projects, you gain practical experience that can be highly valued by employers.
-
Demonstrated Initiative: Pursuing a career in machine learning without a degree showcases your passion, self-motivation, and ability to learn independently, which are highly regarded qualities in the field.
Differences to a Conventional or Academic Path
Choosing a non-conventional path to become a Machine Learning Research Engineer has some key differences compared to a conventional or academic path:
-
Focus on Practical Skills: While academic programs provide a comprehensive theoretical foundation, a non-conventional path emphasizes practical skills and real-world applications.
-
Portfolio vs. Degree: Instead of relying on a degree, professionals without formal education focus on building a strong portfolio of projects to showcase their abilities.
-
Self-Directed Learning: Non-conventional paths require self-directed learning, where individuals take responsibility for their education and skill development.
-
Networking and Connections: Building a network of professionals in the field becomes crucial for learning, mentorship, and career opportunities.
In summary, while a degree can provide a structured path to becoming a Machine Learning Research Engineer, it is possible to achieve this career goal without one. Self-study, practical experience, building a strong portfolio, and networking are essential components of a non-conventional path. It is important to stay motivated, continuously learn, and demonstrate your skills through projects and contributions to the machine learning community.
Quantum Research Scientist, High Performance Simulations
@ Google | Goleta, CA, USA
Full Time Mid-level / Intermediate USD 141K - 202KData Quality Analyst
@ WaFd Bank | Seattle, WA, United States
Full Time Entry-level / Junior USD 76K - 94KPrincipal Machine Learning Systems Engineer
@ Atlassian | Seattle - United States - Seattle, Washington United States; Remote - Remote; San Francisco - United States - San Francisco, California 94104 United States
Full Time Senior-level / Expert USD 190K - 305KGeospatial Data Scientist
@ UK Centre for Ecology & Hydrology | Wallingford, United Kingdom
Full Time GBP 37K - 39KLead Data Scientist
@ Poshmark | US California (Redwood City) - Office, United States
Full Time Senior-level / Expert USD 167K - 237K