Can you become an Applied Machine Learning Scientist without a degree?
An alternative career path to becoming an Applied Machine Learning Scientist with its major challenges, possible benefits, and some ways to hack your way into it.
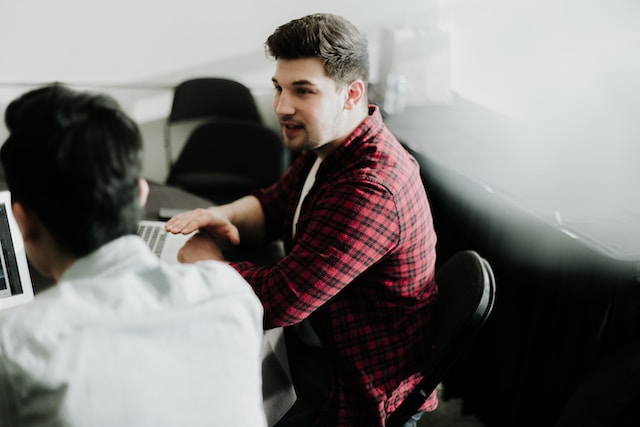
Yes, it is possible to become an Applied Machine Learning Scientist without a degree. While a degree can provide a solid foundation in the field, it is not the only path to a successful career in machine learning. Many employers value practical skills and experience over formal education, especially in the rapidly evolving field of AI/ML.
How to achieve this career goal without a degree
-
Build a strong foundation in mathematics and statistics: Machine learning heavily relies on mathematical concepts such as linear algebra, calculus, probability, and statistics. It is essential to have a solid understanding of these subjects to excel in the field. Online resources, books, and tutorials can help you learn and practice these concepts.
-
Learn programming languages and tools: Proficiency in programming languages like Python and R is crucial for implementing machine learning algorithms. Familiarize yourself with popular libraries and frameworks such as TensorFlow, PyTorch, and scikit-learn. Online courses and tutorials can help you gain practical coding skills.
-
Gain practical experience: Employers often value practical experience and projects more than formal education. Build a portfolio of machine learning projects to showcase your skills. Start with small projects and gradually work on more complex ones. Participate in Kaggle competitions or contribute to open-source projects to gain exposure and demonstrate your abilities.
-
Network and collaborate: Engage with the AI/ML community by attending conferences, meetups, and online forums. Networking can help you connect with professionals in the field, learn from their experiences, and discover potential opportunities. Collaborating on projects with others can also enhance your skills and provide valuable learning experiences.
-
Continuous learning: Stay updated with the latest developments in the field of machine learning. Follow influential researchers, read research papers, and explore new techniques and algorithms. Online courses, tutorials, and MOOCs (Massive Open Online Courses) can also help you expand your knowledge and stay current.
Hacks and advice
-
Online courses and certifications: Enroll in online courses and certifications offered by reputable platforms like Coursera, edX, and Udacity. These courses provide structured learning paths and can help you acquire essential skills and knowledge.
-
Participate in Kaggle competitions: Kaggle is a platform where data scientists and machine learning practitioners compete to solve real-world problems. Participating in Kaggle competitions can help you gain practical experience, learn from others, and showcase your skills to potential employers.
-
Contribute to open-source projects: Contributing to open-source projects not only helps you gain practical experience but also allows you to collaborate with experienced professionals. It can provide valuable learning opportunities and help you establish a strong online presence.
Difficulties, benefits, and differences to a conventional or academic path
Difficulties: Without a degree, you may face challenges in certain job applications that have strict educational requirements. Some employers may prioritize candidates with formal education, especially for research-oriented roles. Additionally, self-learning requires discipline, motivation, and perseverance.
Benefits: Pursuing a career in machine learning without a degree offers several benefits. It allows you to focus on practical skills and gain hands-on experience, which can be highly valued by employers. It also provides flexibility in terms of learning pace, allowing you to tailor your education to your specific needs and interests.
Differences to a conventional or academic path: The conventional academic path provides a structured curriculum and access to expert guidance. It may offer opportunities for research, internships, and networking within academia. However, it can be time-consuming and may not always align with industry demands. The self-taught path allows for a more flexible and tailored learning experience, focusing on practical skills and real-world applications. It can be faster and more cost-effective, but it requires self-motivation and continuous learning.
Senior AI Engineer
@ Lemon.io | Fully Remote
Full Time Freelance Contract Senior-level / Expert USD 57K - 230KSenior Analyst, Information Business Systems and Statistics
@ Canada Mortgage and Housing Corporation | Ottawa
Full Time Senior-level / Expert USD 70K - 87KRRC - Engineering Specialist II-III - Injection Well Analyst - UIC Technical Permitting
@ Texas Comptroller of Public Accounts | Texas-Austin
Full Time Senior-level / Expert USD 54K - 65KDirector, Engineering, ML Compiler
@ Google | Sunnyvale, CA, USA
Full Time Executive-level / Director USD 294K - 414KDevOps Engineer (Hybrid) - 22786
@ HII | Columbia, MD, Maryland, United States
Full Time Mid-level / Intermediate USD 111K - 145K