Data Analyst vs. Data Quality Analyst
Data Analyst vs Data Quality Analyst: A Comprehensive Comparison
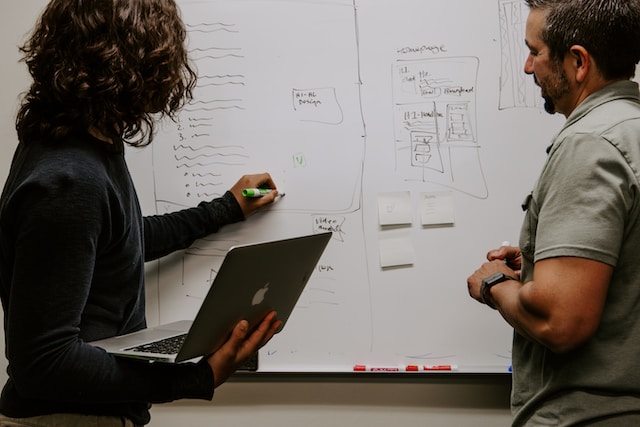
Table of contents
In the rapidly evolving field of data science, understanding the distinctions between various roles is crucial for aspiring professionals. Two prominent positions in this domain are the Data Analyst and the Data quality Analyst. While both roles focus on data, they serve different purposes and require unique skill sets. This article delves into the definitions, responsibilities, required skills, educational backgrounds, tools and software used, common industries, outlooks, and practical tips for getting started in these careers.
Definitions
Data Analyst: A Data Analyst is a professional who collects, processes, and performs statistical analyses on large datasets. Their primary goal is to extract meaningful insights that can inform business decisions and strategies.
Data Quality Analyst: A Data Quality Analyst focuses on ensuring the accuracy, completeness, and reliability of data within an organization. They assess data quality issues and implement processes to improve data integrity, which is essential for effective decision-making.
Responsibilities
Data Analyst Responsibilities
- Collecting and cleaning data from various sources.
- Analyzing data to identify trends, patterns, and insights.
- Creating visualizations and reports to communicate findings.
- Collaborating with stakeholders to understand their data needs.
- Developing and maintaining dashboards for ongoing analysis.
Data Quality Analyst Responsibilities
- Monitoring data quality metrics and identifying discrepancies.
- Conducting data audits to assess data integrity.
- Implementing data quality improvement initiatives.
- Collaborating with IT and Data management teams to resolve data issues.
- Documenting data quality processes and standards.
Required Skills
Data Analyst Skills
- Proficiency in statistical analysis and Data visualization.
- Strong knowledge of SQL and database management.
- Familiarity with programming languages such as Python or R.
- Excellent communication skills for presenting findings.
- Critical thinking and problem-solving abilities.
Data Quality Analyst Skills
- Strong analytical skills to assess data quality.
- Knowledge of Data governance and data management principles.
- Proficiency in data profiling and cleansing techniques.
- Familiarity with data quality tools and methodologies.
- Attention to detail and strong organizational skills.
Educational Backgrounds
Data Analyst Educational Background
- A bachelorโs degree in fields such as Data Science, Statistics, Mathematics, or Computer Science is typically required.
- Advanced degrees (Masterโs or Ph.D.) can enhance job prospects and opportunities for advancement.
Data Quality Analyst Educational Background
- A bachelorโs degree in Information Technology, Data Management, or a related field is common.
- Certifications in data quality management or data governance can be beneficial.
Tools and Software Used
Data Analyst Tools
- Statistical Software: R, Python (Pandas, NumPy)
- Data Visualization Tools: Tableau, Power BI, Google Data Studio
- Database Management: SQL, Microsoft Access
- Spreadsheet Software: Microsoft Excel, Google Sheets
Data Quality Analyst Tools
- Data Quality Tools: Talend, Informatica, Trifacta
- Data Profiling Tools: Apache Griffin, IBM InfoSphere
- Database Management: SQL, Oracle
- ETL Tools: Apache Nifi, Microsoft SSIS
Common Industries
Data Analyst Industries
- Finance and Banking
- Healthcare
- E-commerce and Retail
- Marketing and Advertising
- Technology and Software Development
Data Quality Analyst Industries
- Telecommunications
- Insurance
- Government and Public Sector
- Healthcare
- Financial Services
Outlooks
The demand for both Data Analysts and Data Quality Analysts is expected to grow significantly in the coming years. According to the U.S. Bureau of Labor Statistics, employment for data analysts is projected to grow by 25% from 2020 to 2030, much faster than the average for all occupations. Similarly, as organizations increasingly recognize the importance of data quality, the need for Data Quality Analysts is also on the rise.
Practical Tips for Getting Started
-
Build a Strong Foundation: Start with a solid understanding of statistics and Data analysis techniques. Online courses and certifications can be beneficial.
-
Gain Practical Experience: Internships or entry-level positions in data-related roles can provide valuable hands-on experience.
-
Learn Relevant Tools: Familiarize yourself with the tools and software commonly used in your desired role. Online tutorials and practice projects can help.
-
Network with Professionals: Join data science communities, attend industry conferences, and connect with professionals on platforms like LinkedIn to learn from their experiences.
-
Stay Updated: The field of data science is constantly evolving. Follow industry news, blogs, and Research to stay informed about the latest trends and technologies.
By understanding the differences between Data Analysts and Data Quality Analysts, aspiring professionals can make informed decisions about their career paths and develop the necessary skills to succeed in the data-driven world. Whether you choose to pursue a career as a Data Analyst or a Data Quality Analyst, both roles offer exciting opportunities to make a significant impact in various industries.
Sr. Sales Analyst - Project Manager
@ Avantor | USA-PA Remote, United States
Full Time Senior-level / Expert USD 77K - 128KHuman Research Technologist, Cognitive Aging and Neuroimaging Lab (False Memory Focus)
@ Penn State University | Penn State University Park, United States
Full Time Entry-level / Junior USD 31K - 45KTrader, VP
@ Citi | 388 GREENWICH STREET - TRADING, United States
Full Time Executive-level / Director USD 200K - 250KSenior Quantitative Analyst, Vice President
@ State Street | Boston, Massachusetts, United States
Full Time Senior-level / Expert USD 120K - 187KAVP, Credit Model Development
@ Synchrony | Stamford Site, United States
Full Time Executive-level / Director USD 110K - 185K