Data Engineer vs. Research Scientist
Data Engineer vs Research Scientist: A Detailed Comparison
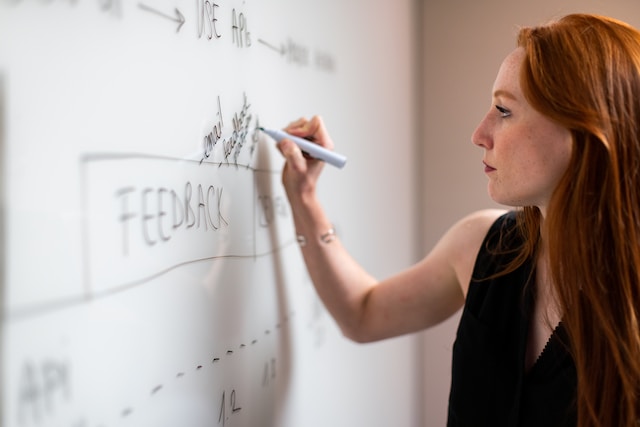
Table of contents
In the rapidly evolving fields of data science and Machine Learning, two prominent roles have emerged: Data Engineer and Research Scientist. While both positions are integral to the data ecosystem, they serve distinct purposes and require different skill sets. This article delves into the definitions, responsibilities, required skills, educational backgrounds, tools and software used, common industries, outlooks, and practical tips for getting started in each role.
Definitions
Data Engineer: A Data Engineer is primarily responsible for designing, building, and maintaining the infrastructure and Architecture that allow for the collection, storage, and processing of data. They ensure that data flows seamlessly from various sources to data warehouses and analytics tools, enabling organizations to make data-driven decisions.
Research Scientist: A Research Scientist in the context of data science focuses on developing new algorithms, models, and methodologies to solve complex problems. They conduct experiments, analyze data, and publish findings to advance the field of machine learning and artificial intelligence.
Responsibilities
Data Engineer
- Design and implement Data pipelines for data collection and processing.
- Develop and maintain data architectures, including databases and data warehouses.
- Ensure Data quality and integrity through validation and cleansing processes.
- Collaborate with data scientists and analysts to understand data needs.
- Optimize data storage and retrieval for performance and scalability.
Research Scientist
- Conduct experiments to test hypotheses and validate models.
- Develop new algorithms and methodologies for Data analysis.
- Analyze large datasets to extract insights and inform decision-making.
- Publish research findings in academic journals and conferences.
- Collaborate with cross-functional teams to apply research in practical applications.
Required Skills
Data Engineer
- Proficiency in programming languages such as Python, Java, or Scala.
- Strong understanding of database management systems (SQL and NoSQL).
- Experience with data warehousing solutions (e.g., Amazon Redshift, Google BigQuery).
- Knowledge of ETL (Extract, Transform, Load) processes and tools.
- Familiarity with cloud platforms (e.g., AWS, Azure, Google Cloud).
Research Scientist
- Expertise in statistical analysis and machine learning algorithms.
- Proficiency in programming languages such as Python or R.
- Strong analytical and problem-solving skills.
- Experience with Data visualization tools (e.g., Matplotlib, Seaborn).
- Ability to communicate complex concepts to non-technical stakeholders.
Educational Backgrounds
Data Engineer
- Bachelorβs degree in Computer Science, Information Technology, or a related field.
- Advanced degrees (Masterβs or Ph.D.) are beneficial but not always required.
- Certifications in data Engineering or cloud technologies can enhance job prospects.
Research Scientist
- Ph.D. in Computer Science, Statistics, Mathematics, or a related field is often required.
- Masterβs degrees may be acceptable for some positions, especially in industry.
- Continuous learning through workshops, conferences, and online courses is essential.
Tools and Software Used
Data Engineer
- Data processing frameworks (e.g., Apache Spark, Apache Kafka).
- Database management systems (e.g., MySQL, MongoDB, PostgreSQL).
- ETL tools (e.g., Apache NiFi, Talend).
- Cloud services (e.g., AWS Glue, Azure Data Factory).
Research Scientist
- Machine learning libraries (e.g., TensorFlow, PyTorch, Scikit-learn).
- Statistical analysis software (e.g., R, SAS).
- Data visualization tools (e.g., Tableau, Power BI).
- Version control systems (e.g., Git) for collaborative research.
Common Industries
Data Engineer
- Technology and software development.
- Financial services and Banking.
- E-commerce and retail.
- Healthcare and pharmaceuticals.
- Telecommunications.
Research Scientist
- Academia and research institutions.
- Technology companies (especially in AI and machine learning).
- Government and public sector research organizations.
- Healthcare and biotech firms.
- Automotive and Robotics industries.
Outlooks
The demand for both Data Engineers and Research Scientists is expected to grow significantly in the coming years. According to the U.S. Bureau of Labor Statistics, employment for data-related roles is projected to grow much faster than the average for all occupations. As organizations increasingly rely on data-driven insights, the need for skilled professionals in both areas will continue to rise.
Practical Tips for Getting Started
For Aspiring Data Engineers
- Learn Programming: Start with Python or Java, focusing on data manipulation and processing.
- Understand Databases: Gain hands-on experience with SQL and NoSQL databases.
- Build Projects: Create personal projects that involve data Pipelines and ETL processes.
- Get Certified: Consider certifications in cloud platforms or data engineering to enhance your resume.
- Network: Join data engineering communities and attend meetups to connect with professionals in the field.
For Aspiring Research Scientists
- Pursue Advanced Education: Consider enrolling in a Ph.D. program focused on machine learning or statistics.
- Engage in Research: Participate in research projects during your studies to gain practical experience.
- Stay Updated: Follow the latest research papers and trends in machine learning and AI.
- Publish Your Work: Aim to publish your findings in reputable journals or present at conferences.
- Collaborate: Work with interdisciplinary teams to broaden your understanding and application of research.
In conclusion, while Data Engineers and Research Scientists both play crucial roles in the data landscape, their responsibilities, skills, and career paths differ significantly. Understanding these differences can help aspiring professionals make informed decisions about their careers in the data science field.
Senior AI Engineer
@ Lemon.io | Fully Remote
Full Time Freelance Contract Senior-level / Expert USD 57K - 230KDirector, Consult Partner - Manufacturing / AI
@ Kyndryl | US152580 New York (US152580) One Vanderbilt, United States
Full Time Executive-level / Director USD 151K - 327KDirector, Consult Partner - Utilities / AI
@ Kyndryl | US152580 New York (US152580) One Vanderbilt, United States
Full Time Executive-level / Director USD 151K - 327KBusiness Analyst Intern - Summer 2025
@ CACI International Inc | 2IG WARRENTON VA (TAX JURISDICTION - FAUQUIER COUNTY), United States
Part Time Internship Entry-level / Junior USD 43K - 87KClinical Trials Feasibility Analyst
@ IQVIA | Durham, North Carolina, United States of America
Full Time Mid-level / Intermediate USD 106K - 177K