Data Operations Manager vs. Head of Data Science
Data Operations Manager vs. Head of Data Science: A Comprehensive Comparison
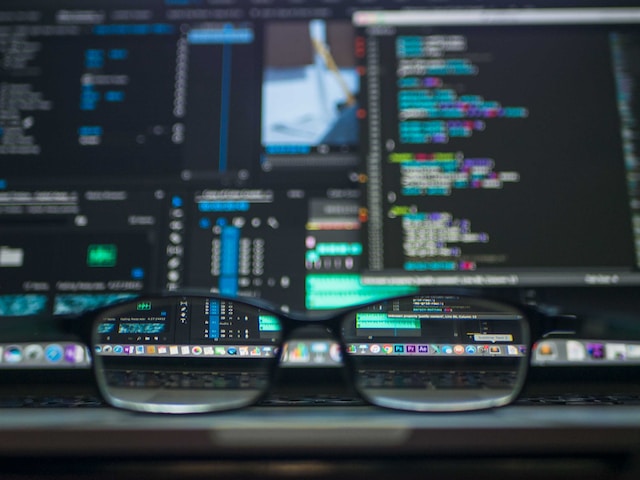
Table of contents
In the rapidly evolving landscape of data-driven decision-making, two pivotal roles have emerged: the Data Operations Manager and the Head of Data Science. While both positions are integral to leveraging data for business success, they serve distinct functions within an organization. This article delves into the definitions, responsibilities, required skills, educational backgrounds, tools and software used, common industries, outlooks, and practical tips for getting started in these roles.
Definitions
Data Operations Manager: A Data Operations Manager oversees the data management processes within an organization. This role focuses on ensuring that data is collected, processed, and utilized efficiently and effectively. They bridge the gap between data engineering and Data Analytics, ensuring that data operations align with business objectives.
Head of Data Science: The Head of Data Science is responsible for leading the data science team and driving the strategic direction of data science initiatives. This role involves developing advanced analytical models, Machine Learning algorithms, and data-driven strategies to solve complex business problems and enhance decision-making.
Responsibilities
Data Operations Manager
- Data governance: Establishing policies and procedures for data management and ensuring compliance with regulations.
- Process Optimization: Streamlining data collection, processing, and storage to improve efficiency.
- Collaboration: Working with IT, data engineers, and analysts to ensure data availability and quality.
- Performance Monitoring: Tracking data operations metrics and implementing improvements based on performance analysis.
- Training and Development: Educating team members on Data management best practices and tools.
Head of Data Science
- Strategic Leadership: Setting the vision and strategy for data science initiatives within the organization.
- Model Development: Leading the design and implementation of predictive models and machine learning algorithms.
- Team Management: Recruiting, mentoring, and managing a team of data scientists and analysts.
- Stakeholder Engagement: Collaborating with business leaders to identify data-driven opportunities and communicate findings.
- Research and Innovation: Staying abreast of industry trends and emerging technologies to drive innovation in data science.
Required Skills
Data Operations Manager
- Data Management: Proficiency in data governance, Data quality, and data lifecycle management.
- Analytical Skills: Ability to analyze data processes and identify areas for improvement.
- Project Management: Strong organizational skills to manage multiple projects and deadlines.
- Communication: Excellent verbal and written communication skills to liaise with technical and non-technical stakeholders.
- Technical Proficiency: Familiarity with Data Warehousing, ETL processes, and database management systems.
Head of Data Science
- Statistical Analysis: Expertise in statistical methods and Data analysis techniques.
- Machine Learning: Proficiency in machine learning algorithms and frameworks.
- Programming Skills: Strong coding skills in languages such as Python, R, or Scala.
- Business Acumen: Understanding of business operations and the ability to translate data insights into actionable strategies.
- Leadership: Strong leadership and team management skills to guide a diverse team of data professionals.
Educational Backgrounds
Data Operations Manager
- Bachelorβs Degree: Typically requires a degree in data science, information technology, Computer Science, or a related field.
- Certifications: Relevant certifications in data management, project management, or data governance can enhance qualifications.
Head of Data Science
- Masterβs or Ph.D.: Often requires an advanced degree in data science, Statistics, computer science, or a related quantitative field.
- Specialized Training: Additional training in machine learning, artificial intelligence, or Big Data analytics is beneficial.
Tools and Software Used
Data Operations Manager
- Data Management Tools: Tools like Talend, Informatica, or Apache NiFi for data integration and ETL processes.
- Database Management Systems: Proficiency in SQL databases (e.g., MySQL, PostgreSQL) and NoSQL databases (e.g., MongoDB).
- Data visualization: Familiarity with tools like Tableau or Power BI for reporting and visualization.
Head of Data Science
- Programming Languages: Proficient in Python, R, or Scala for data analysis and model development.
- Machine Learning Frameworks: Experience with TensorFlow, PyTorch, or Scikit-learn for building machine learning models.
- Big Data Technologies: Knowledge of Hadoop, Spark, or Apache Kafka for handling large datasets.
Common Industries
Data Operations Manager
- Finance: Managing data for risk assessment and compliance.
- Healthcare: Ensuring data integrity for patient records and research.
- Retail: Optimizing data processes for inventory management and customer insights.
Head of Data Science
- Technology: Driving innovation through data analytics and machine learning.
- E-commerce: Enhancing customer experience through personalized recommendations.
- Telecommunications: Analyzing customer data to improve service delivery and retention.
Outlooks
The demand for both Data Operations Managers and Heads of Data Science is on the rise as organizations increasingly rely on data to drive decision-making. According to the U.S. Bureau of Labor Statistics, employment for data-related roles is expected to grow significantly over the next decade, with data science roles projected to grow by 31% from 2019 to 2029. This growth reflects the critical importance of data in various sectors, making both roles essential for organizational success.
Practical Tips for Getting Started
- Gain Relevant Experience: Start in entry-level data roles, such as data analyst or data engineer, to build foundational skills.
- Pursue Education: Consider obtaining a degree or certifications in data science, data management, or related fields.
- Network: Join professional organizations, attend industry conferences, and connect with professionals in the field to expand your network.
- Stay Updated: Keep abreast of the latest trends and technologies in data science and data management through online courses, webinars, and industry publications.
- Develop Soft Skills: Focus on enhancing communication, leadership, and project management skills, as these are crucial for both roles.
In conclusion, while the Data Operations Manager and Head of Data Science roles share a common goal of leveraging data for business success, they differ significantly in their responsibilities, required skills, and educational backgrounds. Understanding these differences can help aspiring professionals choose the right path in the dynamic field of data science and operations.
PhD Positions in Data Science
@ Munich School for Data Science (MUDS) | Munich, Germany
Full Time Entry-level / Junior EUR 45K - 55KCloud Engineer - Bioinformatics
@ Biogen | Cambridge, MA, United States
Full Time USD 115K - 150KSr Staff Engineer Software (Internet Security)
@ Palo Alto Networks | Santa Clara, CA, United States
Full Time Entry-level / Junior USD 126K - 204KSr Principal Engineer Software (Gen AI)
@ Palo Alto Networks | Santa Clara, CA, United States
Full Time Senior-level / Expert USD 170K - 270KSr. Data Engineer
@ Visa | Bellevue, WA, United States
Full Time Senior-level / Expert USD 116K - 164K