Data Science Engineer vs. Finance Data Analyst
Data Science Engineer vs Finance Data Analyst: A Comprehensive Comparison
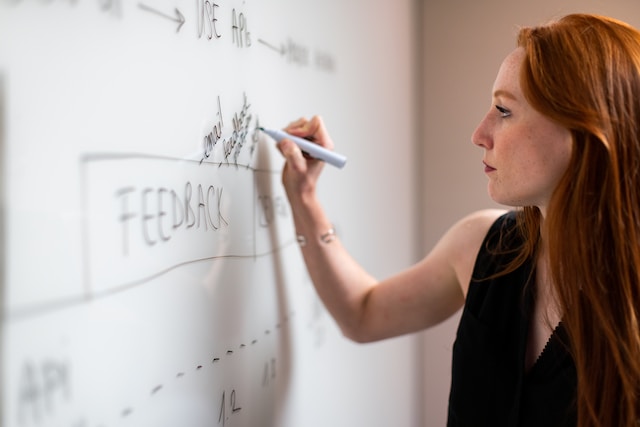
Table of contents
In the rapidly evolving landscape of data-driven decision-making, two prominent roles have emerged: Data Science Engineer and Finance Data Analyst. While both positions leverage data to derive insights, they differ significantly in their responsibilities, required skills, and career trajectories. This article delves into the nuances of each role, providing a detailed comparison to help aspiring professionals make informed career choices.
Definitions
Data Science Engineer: A Data Science Engineer is a technical expert who focuses on designing, building, and maintaining data systems and infrastructure. They work on Data pipelines, algorithms, and machine learning models to enable data-driven solutions across various domains.
Finance Data Analyst: A Finance Data Analyst specializes in analyzing financial data to support business decisions. They interpret financial metrics, create reports, and provide insights that help organizations optimize their financial performance and strategy.
Responsibilities
Data Science Engineer
- Data Pipeline Development: Design and implement robust data Pipelines for data collection, storage, and processing.
- Model Development: Build and optimize Machine Learning models to solve complex business problems.
- Collaboration: Work closely with data scientists, analysts, and stakeholders to understand data requirements and deliver solutions.
- Data quality Assurance: Ensure data integrity and quality through rigorous testing and validation processes.
- Scalability: Develop scalable data architectures that can handle large volumes of data efficiently.
Finance Data Analyst
- Data analysis: Analyze financial data to identify trends, variances, and anomalies.
- Reporting: Create detailed financial reports and dashboards for stakeholders to facilitate decision-making.
- Forecasting: Develop financial models to predict future performance based on historical data.
- Risk Assessment: Evaluate financial risks and opportunities to guide investment strategies.
- Collaboration: Work with finance teams and management to provide insights that drive business strategy.
Required Skills
Data Science Engineer
- Programming Languages: Proficiency in languages such as Python, R, and SQL.
- Machine Learning: Strong understanding of machine learning algorithms and frameworks (e.g., TensorFlow, PyTorch).
- Data Engineering: Knowledge of data warehousing, ETL processes, and Big Data technologies (e.g., Hadoop, Spark).
- Statistical Analysis: Ability to apply statistical methods to analyze and interpret data.
- Cloud Computing: Familiarity with cloud platforms (e.g., AWS, Azure) for deploying data solutions.
Finance Data Analyst
- Financial Acumen: Strong understanding of financial principles, accounting, and investment analysis.
- Data visualization: Proficiency in tools like Tableau, Power BI, or Excel for creating visual reports.
- Analytical Skills: Ability to analyze complex datasets and derive actionable insights.
- Communication: Strong verbal and written communication skills to present findings to non-technical stakeholders.
- Excel Mastery: Advanced skills in Excel for financial modeling and analysis.
Educational Backgrounds
Data Science Engineer
- Degree: Typically holds a degree in Computer Science, Data Science, Statistics, or a related field.
- Certifications: Relevant certifications in data Engineering, machine learning, or cloud computing can enhance job prospects.
Finance Data Analyst
- Degree: Often holds a degree in Finance, Economics, Business Administration, or a related field.
- Certifications: Professional certifications such as CFA (Chartered Financial Analyst) or CPA (Certified Public Accountant) can be beneficial.
Tools and Software Used
Data Science Engineer
- Programming Languages: Python, R, SQL
- Machine Learning Frameworks: TensorFlow, Keras, Scikit-learn
- Data Processing Tools: Apache Spark, Hadoop
- Database Management: MySQL, PostgreSQL, MongoDB
- Cloud Services: AWS, Google Cloud Platform, Microsoft Azure
Finance Data Analyst
- Data Analysis Tools: Excel, R, Python
- Visualization Software: Tableau, Power BI, QlikView
- Financial Modeling Tools: Bloomberg Terminal, FactSet
- Database Management: SQL, Oracle
- Statistical Software: SAS, SPSS
Common Industries
Data Science Engineer
- Technology
- Healthcare
- E-commerce
- Finance
- Telecommunications
Finance Data Analyst
- Banking
- Investment Firms
- Insurance
- Corporate Finance
- Consulting
Outlooks
Data Science Engineer
The demand for Data Science Engineers is expected to grow significantly as organizations increasingly rely on data-driven solutions. According to the U.S. Bureau of Labor Statistics, employment in data-related fields is projected to grow by 31% from 2019 to 2029, much faster than the average for all occupations.
Finance Data Analyst
The outlook for Finance Data Analysts remains strong, particularly as businesses seek to optimize their financial performance. The Bureau of Labor Statistics projects a 5% growth in employment for financial analysts from 2019 to 2029, driven by the need for financial expertise in various sectors.
Practical Tips for Getting Started
- Identify Your Interest: Determine whether you are more inclined towards technical data engineering or financial analysis.
- Build a Strong Foundation: Acquire relevant educational qualifications and certifications to enhance your knowledge and skills.
- Gain Practical Experience: Seek internships or entry-level positions to gain hands-on experience in your chosen field.
- Network: Connect with professionals in the industry through networking events, online forums, and social media platforms like LinkedIn.
- Stay Updated: Keep abreast of the latest trends and technologies in data science and finance to remain competitive in the job market.
In conclusion, both Data Science Engineers and Finance Data Analysts play crucial roles in leveraging data for decision-making. By understanding the differences in responsibilities, skills, and career paths, aspiring professionals can make informed choices that align with their interests and career goals. Whether you choose to delve into the technical realm of data engineering or the analytical world of finance, both paths offer rewarding opportunities in todayβs data-driven economy.
Senior Machine Learning Engineer
@ Freeform | Los Angeles, CA (On-site)
Full Time Senior-level / Expert USD 140K - 250KStaff Applied Research Scientist
@ ServiceNow | Santa Clara, California , United States
Full Time Senior-level / Expert USD 173K - 303KSenior Solutions Architect - Data Analytics
@ Boeing | USA - Hazelwood, MO, United States
Full Time Senior-level / Expert USD 190K+2025 University Grad - Process Engineer (PhD)
@ Applied Materials | Santa Clara,CA, United States
Full Time USD 124K - 171KSenior Backend Software Engineer III (E3)
@ Applied Materials | Chandler,AZ, United States
Full Time Senior-level / Expert USD 132K - 181K